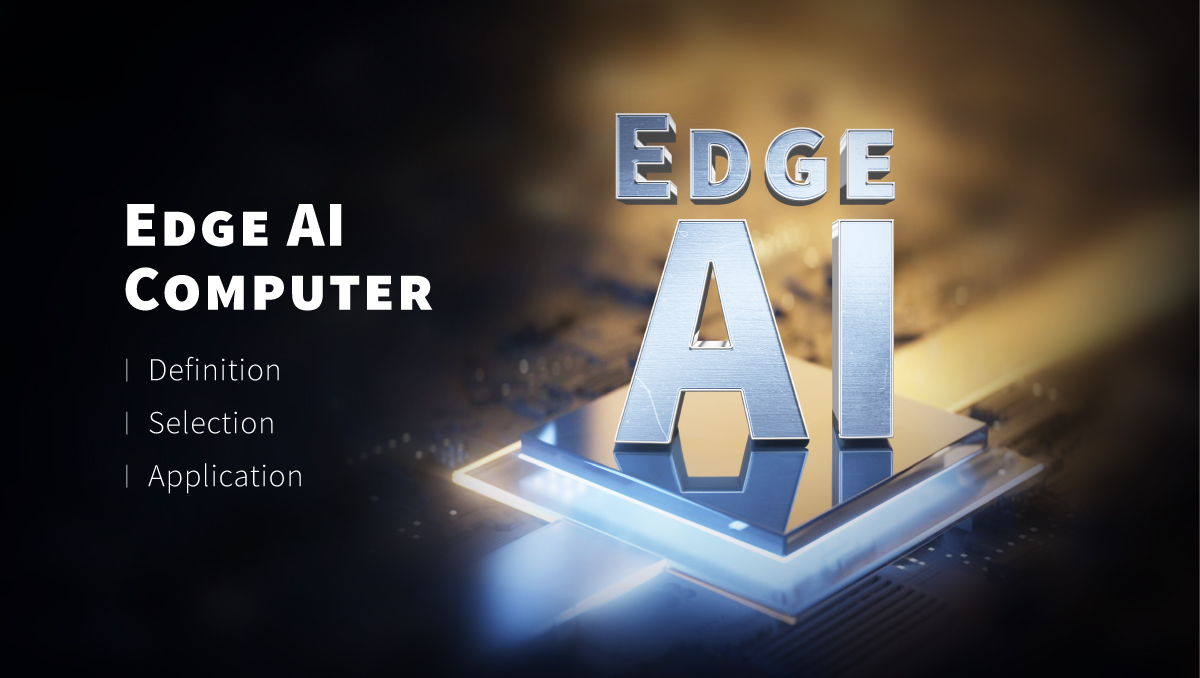
What is an Edge AI computer ? Discover the definition, selection, and applications
Edge AI brings the power of AI from the data center to edge computers. This shift promises to enable real-time analysis and action by bringing analysis closer to the source and eliminating slow data roundtrips. Beyond the promised benefits, there are some important factors to consider, especially in terms of edge computer performance, when making this shift. This article sheds light on all these factors to give you a complete picture of this emerging technological shift.
What is Edge AI computer?
In traditional AI deployments, the edge computer sends all data to a powerful server for model training and predictions. The server must implement specific network security protocols to enable secure connections for users at many locations and allow access to AI models.
Edge AI brings AI capabilities to edge computing. It takes powerful AI models and algorithms from the data center and places them on the edge computer. Moving AI processing to the edge computer enables fast data collection, real-time processing, and machine learning close to the data source, eliminating the transmission of large amounts of data to distant cloud servers. The fusion of these technologies accelerates processing time, improves response times, reduces bandwidth demands, and bolsters security throughout.
In the workflow described above, an Edge AI computer is responsible for data analysis and intelligent judgment at the edge. They must have the environmental adaptability and network capabilities of a regular edge computer but with much higher performance to meet the complex AI processing demands. From factories, hospitals, and parking lots to street lights and charging piles, Edge AI computers can realize real-time data processing and decision-making and improve operational efficiency through smart technology.
Key considerations for an Edge AI computer
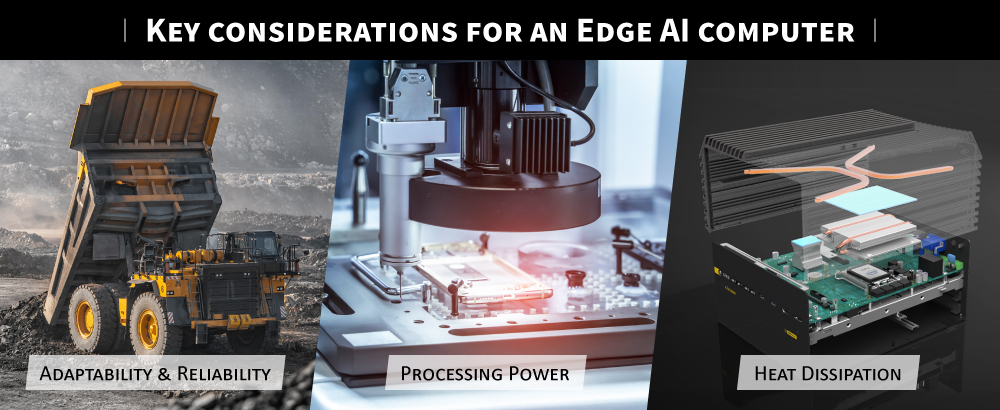
Environmental adaptability and reliability
Industrial sites, the typical location of Edge AI computers, place much more rigorous environmental demands on computers compared to the relative comfort of data centers or offices. These unpredictable environments, including remote suburbs, humid and low-temperature fresh food factories, or continuously vibrating train carriages, test the computer’s ability to operate continuously and stably in harsh environments, making high reliability non-negotiable for Edge AI computers. Edge AI computers must overcome the basic challenges of temperature, humidity, and vibration and deal with more severe challenges like unstable voltages, electromagnetic interference, or potential security risks that may appear in the environment. Various industrial-grade protections, such as overcurrent, overvoltage, and ESD protection have become standard. UL safety certification and meeting EMC standards for industrial environments (such as EN 61000-6-2 and EN 61000-6-4) are also factors worth considering when choosing an Edge AI computer. These certifications will ensure the reliability and safety of the equipment in various environments.
Processing power
Edge AI emphasizes the local processing of machine learning and AI models rather than remote processing on a cloud server, so the edge computer’s computing power is critical. The three core elements of AI include computing power, algorithms, and data. Users can determine the computing power required for image reasoning or judgment based on the amount of computing, data volume, and processing speed.
For processing basic Edge AI applications, the computing power of the CPU may be sufficient, especially as Intel continues to improve CPU performance and integrate more GPU computing capabilities, coupled with the increase in neural processing units (NPUs), many inference applications can be completed without additional accelerators. But for more sophisticated and complex industrial applications, you need to choose mid- to high-end Edge AI computers equipped with independent GPU graphics cards or dedicated accelerators.
Currently, there are three main types of GPU cards on the market: SoM, MXM, and PEG. The SoM (System on Module) GPU is a small-sized GPU with low power consumption specifically designed for embedded systems and typically used in applications that require high performance and low power consumption. The MXM (Mobile PCI Express Module) GPU is a flexible and easily upgradeable pluggable GPU module widely used in industrial computers. The PEG (PCI Express Graphics) GPU is a traditional graphics card, usually inserted into the motherboard’s PCIe slot of the motherboard, providing the most powerful graphics processing capabilities and is suitable for applications that require extremely high computing performance, such as high-frequency image processing and deep learning. Users can choose the most appropriate solution based on specific task requirements.
Heat dissipation
CPU and GPU temperatures in Edge AI computers rise rapidly because of extended continuous operation. Without excellent heat dissipation technology to keep the system cool, it will overheat and automatically throttle performance by reducing the clock frequency, impairing the computing efficiency. Because of these factors, the cooling technology used for Edge AI computers has also become a critical factor.
Cincoze’s flagship Edge AI computer, the GP-3000, has passive cooling technology to keep the system as cool as possible and an external fan that provides additional airflow during extended operation. The shell combines thermally conductive copper and heat dissipating aluminum formed with an exclusive heat dissipation pattern. From the start, we’ve considered the dust and impurities often found in the industrial environment and designed our patented dustproof cooling mechanism (Patent No. I778522) that concentrates the airflow of the fan to remove heat more effectively and prevents dust and dirt from entering the system, thereby extending the service life of the equipment.
Common applications of Edge AI computers
Manufacturing – quality control and defect detection
Quality control and defect detection are typical applications of Edge AI in manufacturing. Edge AI computers equipped with high-performance CPUs and GPUs can process high-definition images from high-resolution cameras in real time and perform intelligent analysis by running pre-trained AI models to identify surface defects, dimensional deviations, or shape abnormalities that are difficult to detect with the naked eye. Production abnormalities or defects are immediately noted and provided to operators or automated systems for subsequent repairs or adjustment of production parameters. This shortens the inspection time and reduces the defect rate, strengthening corporate competitiveness and customer satisfaction through effective quality control.
Case Study: A well-known automation equipment manufacturer in Asia Pacific used the Cincoze GP-3000 equipped with Intel Core i CPU and NVIDIA GeForce RTX 3070 GPU card as a PCBA defect detection system. The company used AOI and deep learning software to accurately detect and mark PCBA defects, such as material surface defects, welding defects, and misplaced or missing components. Normal and defective products are categorized completely automatically, greatly improving detection efficiency and yield.
Transportation – intelligent traffic light control system
Building smart cities is the goal of many governments around the world. Intelligent traffic light control systems are an important part of that goal, providing citizens with a smoother and more environmentally friendly travel experience. Traffic control centers can analyze real-time traffic data, predict vehicle behavior with machine learning algorithms, and adjust the timing of traffic signals. These measures relieve congestion by shortening waiting times at red lights, decreasing wasted fuel while sitting idle, and reducing the city’s carbon emissions.
Case Study: The Environmental Engineering Research Institute of a top European university developed an intelligent traffic light control system to improve the traffic congestion in their city. This system connects peripheral monitoring equipment and detection radars through Edge AI computers. These systems process relevant traffic data in real time and perform intelligent analysis through pre-trained AI models to optimize the control of traffic signals.
Energy – smart drilling in offshore oilfields
Offshore drilling is complex and potentially dangerous. Smart drilling systems improve the efficiency and safety of drilling operations by using Edge AI to perform instant analysis and decision-making on large amounts of data. The Edge AI computer instantly processes data from geological, pressure, temperature, and other sensors, then uses AI algorithms to analyze and optimize drilling operations or perform predictive equipment maintenance. These systems shorten drilling time, prevent potential safety risks, and ensure the stability and safety of drilling operations.
Case Study: A highly recognized oil and gas industry equipment manufacturer in Northern Europe, known for its leading technologies in production, drilling, and processing, used the Cincoze DS-1402 as the Edge AI computer to optimize the efficiency and safety of their smart drilling system and provide better and faster drilling processes.
In-vehicle – police vehicle surveillance system
AI technology has joined the fight against crime, and smart police cars with in-vehicle surveillance have become indispensable for maintaining urban security. Mobile surveillance systems installed in smart police vehicles help curb criminal behavior. They use license plate recognition to read license plates, then run the plates through the traffic management system to quickly identify stolen or problematic vehicles so officers can take immediate action.
Case Study: A European military equipment manufacturer built a smart police vehicle surveillance system with the Cincoze DX-1100. The system can record photographs, video, and the current position of a vehicle, recognize the license plate and run it through the vehicle database to quickly identify suspicious vehicles. These measures improve the police enforcement efficiency of frontline personnel.